AK
akarchmer0@gmail.com -
github -
google scholar -
follow me on X!
I'm a postdoc at
Harvard University, where I participate in the SAFR AI lab led by Seth Neel and Salil Vadhan. I obtained my Ph.D. from
Boston University under the supervision of Ran Canetti in spring of 2024.
I'm interested in machine learning, AI, and theoretical computer science.
I want to help develop the theory of how machine learning really works—can we predict how ML models will perform/act, before we train (or otherwise do surgery on) them?
For instance, some of my recent work contributes to
a better understanding when and why multimodal data is useful for ML.
I am now also working on applied research in ML/AI interpretability, such as methods for data attribution and mechanistic interpretability and model editing.
My Ph.D. thesis was in the area of meta-complexity, and in particular, discovered new relationships between the complexity theory of circuit lower bounds and computational learning theory.
blog
>>>
berkeley cafe museum
>>>
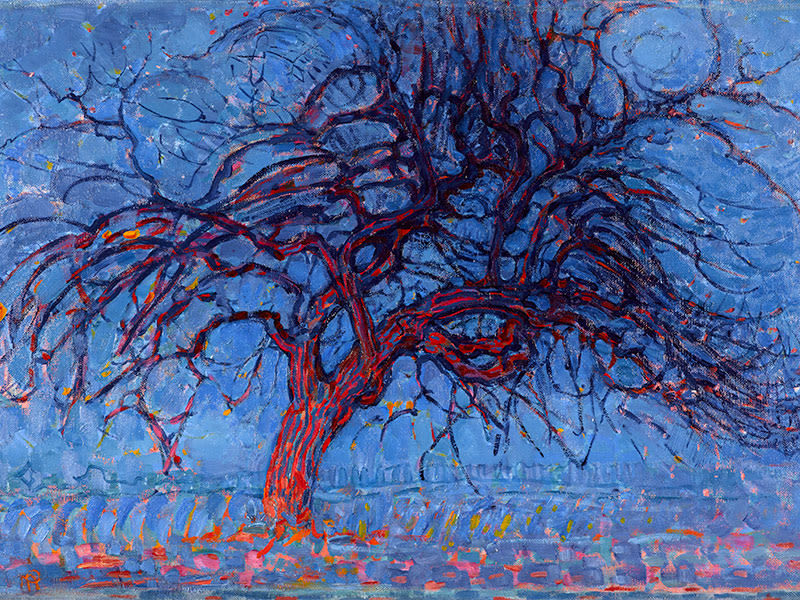
Piet Mondrian, The Red Tree, 1908